Bridging the Gap: Exploring AI’s Role in Design Thinking with academic rigor (in 2023).
By Daniela Marzavan, Founder at Change Darer. Practitioner and academic in the field of Innovation and Design Thinking

Do you prefer fast food made from ingredients conveniently stored, following standard recipes, or would you rather indulge in individually created slow food, carefully crafted from nutritious ingredients you’ve just handpicked?
At Change Darer, we challenge individuals to question the status quo, reframe challenges from their perspective of agency, and apply collective swarm intelligence within interdisciplinary groups to generate implementable solutions. We mostly use tools, methods, and techniques inherent to what is widely known as Design Thinking.
Since January 2023, we have also explored the integration of ChatGPT, an AI assistant, in certain phases of our process.
In this article, I offer anecdotal insights into the juxtaposition of artificial intelligence and academic intelligence in the phases of Understand, Point of View, and Ideate. In the case of the challenge tackled here, the remaining phases heavily rely on human interaction and creativity, to such an extent that we did not even attempt their integration with AI.
Context & Target Group:
In April, we conducted an experiment involving 20 post-doctoral fellows from two prestigious universities specializing in Humanities research. Their yearly symposium aimed to tackle wicked problems, such as diversifying academic science, stimulating cooperation for Planetary Health, communicating Humanities effectively, and fostering living together in a democracy.
What did not work: Analysis in the Understand Phase
These high-level post-doctoral fellows embody repositories of peer-reviewed, sound-proof knowledge, supported by years of interpreting complex data through rigorous methodologies. It was no surprise, when comparing the outcomes of 4 hours of prompting ChatGPT with a 30-minute brainstorming session among these 20 brilliant minds, it became evident that applying ChatGPT in the Understand Phase proved ineffective. The AI-generated responses appeared shallow, just like pre-fabricated political speeches delivered by ill-advised politicians.
What worked: Point of View simplification
The initial challenges, formulated by the academic fellows with their deep knowledge and insights, occasionally suffered from over-intellectualization, demanding slow and repeated reading. Chat GPT managed to distill the main ideas in simple language ranking the challenges according to feasibility and viability. Like a slow cooked dish that will only get appreciation with the right wine and mindset, the initial challenge reformulation would not get appreciation in this fast-paced workshop. The simplification was helpful at this stage.
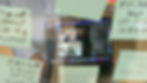
What kind of worked: basic Idea generation
We were dealing with academic researchers who excel at asking profound questions, yet providing operational solutions within time constraints is not their primary role. Interestingly, one group generated a solution similar to the one suggested by ChatGPT, although the human participants demonstrated superior elaboration, prototyping, and storytelling capabilities.
What Prompts we used?
We used prompts mimicking the trend and context analysis: “Please act as an analyst and tell me the most important trends that affect the following challenge:” Second prompt was: Now, give me a PESTEL analysis of this challenge
When the prompts were more challenging and required not only word aggregation and language simulation but also critical analysis, the AI resembled an overconfident, ill-prepared student skilled in the game of “bullshit bingo.”
Initially, it attempted to explain the general concepts, seemingly buying time, before providing shallow and generic answers. For example, it mentioned how the political environment could impact funding accessibility for the given challenge, or how changes in the economy might affect the affordability of solutions. However, the AI failed to provide specific institutions, experts, citations, or a comprehensive analysis of the intricate connections between stakeholders and factors, and their influence on problem-solving. As pages were filled with words, the brain remained empty, just like consuming fast food may fill the stomach but fails to provide nutrients to the body.
In conclusion
While language models like ChatGPT have revolutionized text summarization and stylization, effectively transforming large volumes of data into written form, relying on shallow, anecdotal knowledge sourced from the internet may hinder our capacity to tackle wicked problems, inhibiting deep understanding and critical analysis.
These being said we should not overlook the tremendous opportunities presented by Large Language Models (LLMs) and OpenAI’s technology for our society and the academic world.
In 2018 as a PhD student, I conceptualized an app idea based on Machine Learning. The vision involved an automated “dictionary” that translated complex academic research papers into visually intuitive language and concise videos. This two-step Machine Learning engine would first translate words into icons, producing academic posters, while the second ML-based engine would generate one-minute explanation videos from text and icons. To ensure high-quality outputs, curated peer-reviewed research papers would be handpicked, providing a solid foundation of reliable knowledge.
In 2020, we launched a pivot called “The Health Manager,” during a Covid Hackathon… maybe it’s now the right time for such an App.
Let’s engage in a dialogue, with @OpenAI, to explore the possibilities of creating “brain food” that combines nutritious ingredients with a touch of human insight!